Predictive Analytics: The Future of Digital Marketing
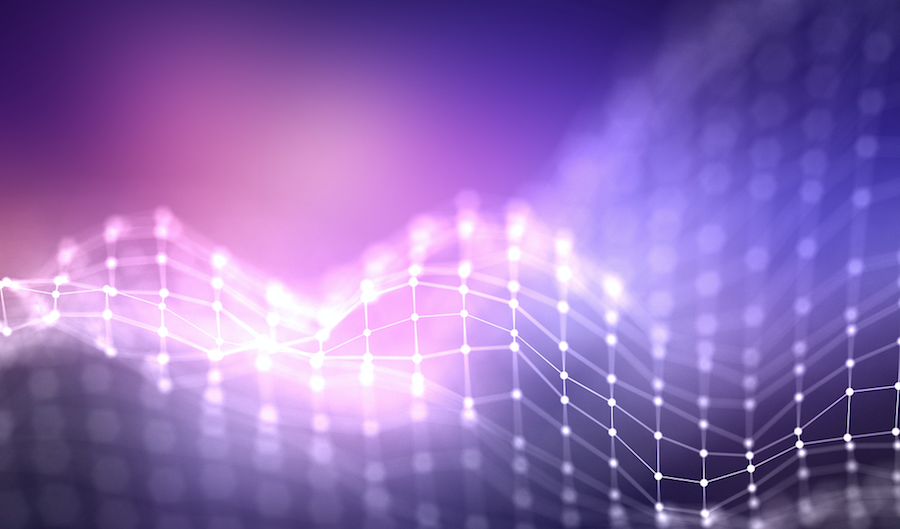
“Yesterday is history, tomorrow is a mystery, and today is a gift… that’s why they call it present.” – Master Oogway
Human beings have always been fascinated by Tomorrow. We have astrologers, psychics and palm readers trying to predict what will happen tomorrow using “super-natural” powers.
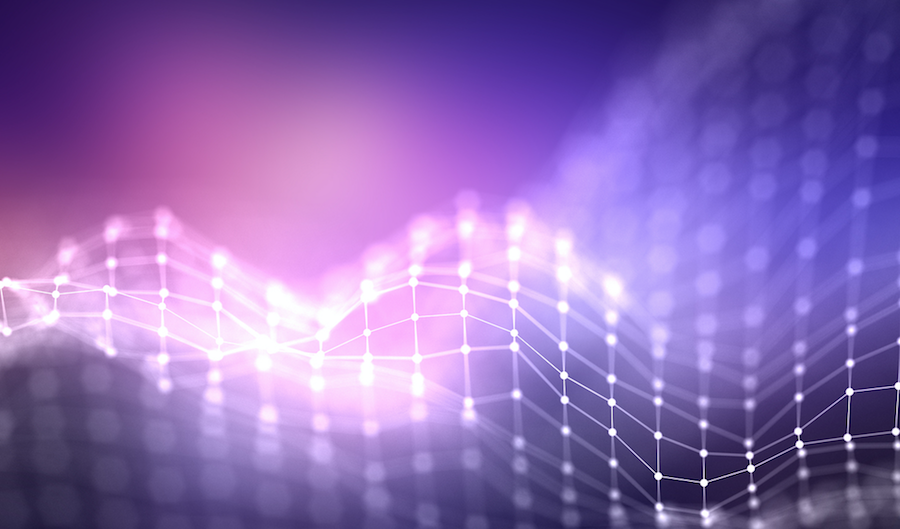
Until recently, most of such prediction efforts have not been positive or consistent, and if someone had predicted something accurate, it has always been more of luck than science. If prediction using super-natural powers were true, most of the millionaires in our world would be the astrologers.
However, as technology impacts every aspect of our lives, it is also creating an impact in predicting the future, with reasonable accuracy. With mathematics, big data and predictive analytics, predictions can be made on the foundation of science. No one can predict the future with 100% accuracy, but a hazy view of what’s going to come is better than blindness any day.
In this article, let us look at how much predictive analytics has evolved and if it has a role to play in the field of digital marketing. Before we dive into predictive analytics for digital marketing, we need to understand how prediction works in the first place, the tools and data we need, and most importantly, its limitations.
How Big Data Helps in Prediction
Until 100 years back, prediction of the future with the use of data was impossible. There were no devices or instruments to store data, let alone process it. Data storage was limited to print and processing the data was limited to our brain’s capacity.
We humans are good with emotions, dealing with other humans and keeping ourselves alive. But our brains were not designed to process huge amount of data.
With the computing revolution, data storage in large volumes for an indefinite period of time is not a problem anymore. According to Moore’s law, computing power (transistors per square inch to be exact) has doubled every year since the invention of micro processors. Storage has also become cheaper.
The phone or computer that you are reading this post on right now definitely has a much higher processing power than what governments and NASA had 50 years back. The cost of per GB of data stored has been decreasing exponentially and when quantum storage disks come into use, the cost of data storage will become negligible.
Before getting too technical into how predictive analytics works, let me try to present how prediction works with data. One of the basic things to remember when it comes to prediction with data is that your prediction accuracy becomes higher with the size of the data. (That’s why big data is important).
No amount of technology or scientific progress can predict the outcome of a single random event – like a coin toss with 100% accuracy. Such a event is truly out of scope, unless we invent a time machine. (Actually, micro-predictive analytics makes it possible to predict on an individual level, with some level of accuracy – this is discussed in the last part of this article).
The bigger the data, higher is the accuracy on a macro level to predict the collective outcome of the events. To explain how prediction accuracy increases with the size of data, let me give you a simple example.
The Roll of a Dice
Imagine a dice. This dice has 6 sides, with numbers from 1 to 6. If you throw the dice 6 times, in an ideal world, we should be getting each number once. But it doesn’t happen that way. 1 may appear twice, 2 not at all, 3 not at all, 4 may be thrice and so on. The randomness is high. That’s the law of probability. This law makes a lot of games interesting!
However, think about throwing the dice 60 times. In an ideal world, each number should appear 10 times, but it doesn’t happen that way in the real world. However, you are bound to get each number at least a few times. There is very little chance that a single number doesn’t even show up once. If I have to make a bet where if I get the number 5 on a dice if I throw it at least 100 times, I would confidently do the bet. The chances are pretty high for a 5 to show up at least once if I roll the dice a 100 times.
Now imagine throwing the dice 6 million times. Every number would be appearing at least 900,000 times. This is getting closer to the ideal state. This is because of the law of large numbers and regression to the mean.
When large numbers are involved, the numbers tend to get closer to the average. Higher the numbers, closer is the data to the averages. To understand this concept with a real experiment, let us take a coin toss, where the outcome can be heads or tails. I am taking a coin toss because it is easier to experiment with.
Understanding ‘Regression to Mean’ with Coin Toss
If you toss a coin once, you cannot say if it will be Heads or Tails. There is a 50/50 chance. The result will be either heads or tails. Statistically, that would be Heads 100% and Tails 0%, or Heads 0% and Tails 100%.
However, if you toss the coin 10 times, you can be pretty sure you will get heads at least a few times. May be 3 times heads and 7 times tails.
If you toss the coin 100 times, you will get Heads and Tails which is closer to 50% each. May be 40 times heads and 60 times tails. If you toss the coin 1 million times, each one would show up like 48% and 52%.
With more number of tries, you would observe that the results are regressing towards the mean. Larger the sampling size, closer are the results to the mean.
If you think about every coin toss in this world ever made from the beginning of time, the number of times heads and tails appeared across every currency would be more like 49.99% and 50.01%.
To test this out, let us head to this website called “Virtual Coin Toss”. This website does a random coin toss every time you Flip.
For the first flip, there is a 50% chance that it will give me heads and a 50% chance that it will give me tails.
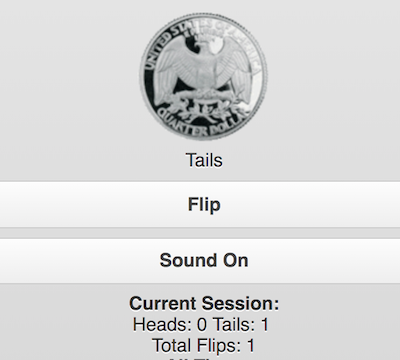
So it gave me Tails.
Since we flipped it only once, the result is way off from the average. The prediction is that there is a 50/50 chance of heads and tails. But here, the tails is at 100% and heads is at 0%.
Now, what if I flip it 10 times?
The regression towards the mean will start happening. I am pretty sure I will get heads at least a few times. Pretty sure it wouldn’t be 0.
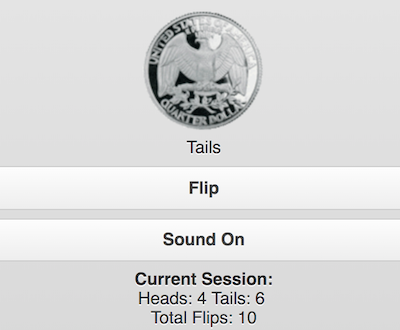
We got heads 4 times and tails 6 times. That’s 40% and 60%. This is closer to the 50/50 that is part of the system.
Now let’s do it 100 times.
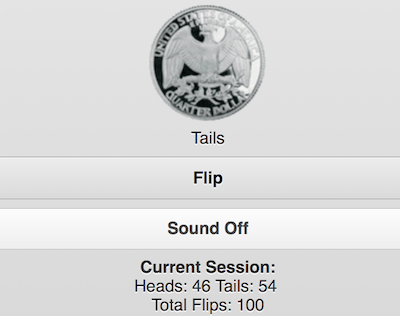
Heads came up 46 times and Tails came up 54 times. That’s 46% and 54%. Each value is closer to 50% this time.
Now this website also shows the lifetime flips on the site. This is all the flips, done by every user since the inception of this site.
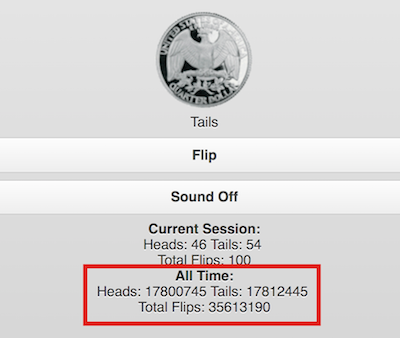
The total flips is 35.6 Million. With such a large number, the total Heads and total Tiles would be very close to 50%. The Heads are at 17.8 Million and Tails are at 17.8 Million. If you look at the exact number, for Heads its 49.98% and for Tails its 50.02%.
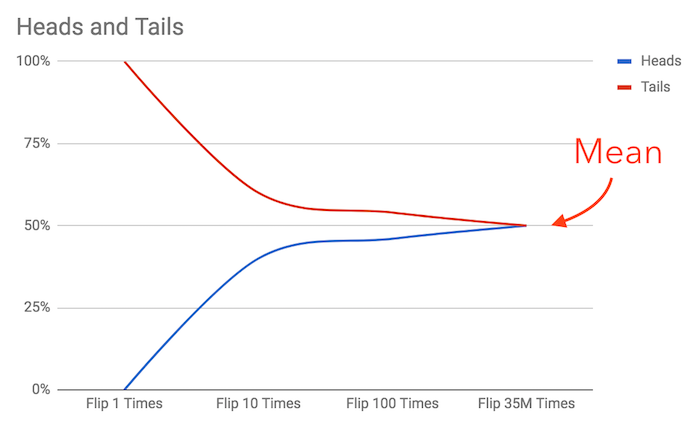
Both Heads and Tails are very close to 50%, as expected. You can see regression to the mean in action in the above chart.

As we go for higher number of flips, both the lines tend to come towards the mean, that’s 50%. It is almost magical because there are no exceptions. The regression to the mean happens always, without failure. The curve never deviates away as the number of tries go up, it always tends to go towards the mean.
Regression to the Mean in Nature
There is no way to predict if the kid will be a male or female before it is conceived. But in a world with billions of people, you can be pretty sure that if 1,00,000 kids are born in a week, approximately 50% will be male and 50% will be female.
Nature has made the randomness in such a way that with large numbers, regression to the mean will take care of the gender balance among mammals. Most of the mathematicians have derived such inspiration from nature.
Predicting Clicks, Leads and Sales in a Funnel
With the law of large numbers and regression towards the mean we can predict clicks, leads and sales in digital marketing. An individual internet user clicking on an ad is a random event, but when we have enough people doing it, we can predict the future, with the data from the past.
If I share a blog article on my Facebook wall, 1-3% of the people who view it will click on it. This has been a repetitive trend. Based on this data, I can be pretty sure that if 10,000 people are going to see my post, at least 100 people are going to click on it. If every blog post that I make doesn’t follow these rules, I might not be motivated to write such a long blog post in the first place.
The same goes for leads and sales in the funnel. Marketers can predict the numbers based on previous data. The cost per lead, cost per sale and metrics involving investment can be predicted with reasonable confidence before one puts their hard earned money into advertising. That’s how one can grow their business funnel.
The true power of predictive analytics comes into play when we get into micro prediction: predicting individual user behaviour.
Micro and Macro Predictive Analytics
The kind of predictive analytics that we have discussed until now is on a macro level. This is closer to forecasting than prediction. Forecasting works on a larger scale and gives directions to future trends based on past data.
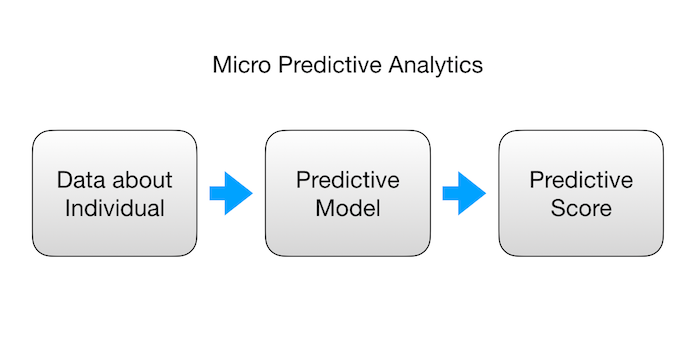
If we predict that 2% of a website’s visitors click on a specific ad, it is a forecast. But do we know who these individuals are? And can we predict that certain individuals will make a purchase after clicking on an ad?
If we do not have much data about the people who click, then we cannot predict what they will do next. However, if we have more data points about each internet user, we can start predicting their behaviour based on sample sets taken from the past.
For example, I have observed a trend that most of the people who buy my SEO course have also purchased my AdWords course. However, it is not true in the case of Facebook Ads Course. So if there are a group of individuals who have purchased my SEO course but not my AdWords course, then I can approach these people and give an offer for the AdWords course. I might not do the same for the people who have purchased the Facebook Ads Course but not the AdWords course.
Such pattern recognition and prediction is very useful in lead scoring. A lot of sales CRM tools such as HubSpot, Marketo and Lattice Engines are taking predictive lead scoring seriously. HubSpot has also opened up their integration to Infer, a predictive lead scoring tool.
According to Infer:
“Infer matches data from your HubSpot portal to thousands of signals from across the web to calculate a score that tells you which leads are most likely to convert or have the biggest revenue impact. This is done using advanced machine learning using data about historical winners and losers captured in HubSpot. Infer takes all of the rich data you collect in HubSpot and distills it down to a single number that the sales team can use to sort, prioritize, and determine who is worth following up with.”
I have not used Infer personally yet, but it is interesting and promising to see new startups working in the area of predictive analytics for marketing and sales. Infer also has integration with SalesForce. You can watch this YouTube video about Infer to know more.
Target’s Prediction of Pregnant Shoppers
Target is the second largest discount store in the US and just next to Walmart in terms of revenues. It has 1,800+ stores with annual revenues of 70+ Billion Dollars. Target’s application of predictive analytics to find out which of their customers are pregnant is an interesting case study.
A small percentage of people who shopped in Target stores opted in to share their personal information. This included people who were pregnant. Target took data of all the female shoppers who said they were pregnant and observed their purchase history. This included medicinal supplements such a folic acid and cocoa butter cream which helped pregnant woman prevent stretch marks on their skin.
Based on this data, if target observers that a female shopper (that has not opted in to share personal information) purchases all these items, then there is a good chance that they are pregnant.
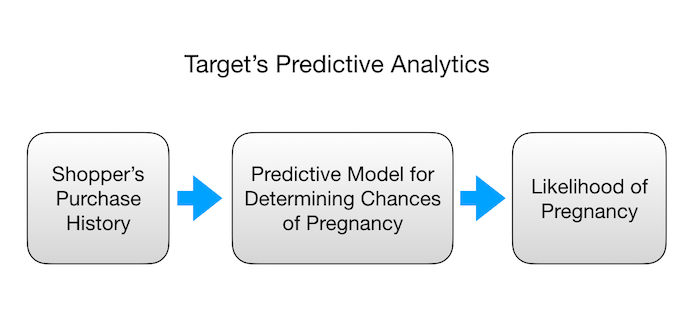
Target takes into account at least 10 different items that are purchased and scores each buyer on how likely they are to be pregnant. If the buyer passes a certain score, then Target can predict with reasonable accuracy that they are pregnant. Target then sends coupons and offers in the mail that are crafted for a pregnant woman’s needs in the second trimester.
Of course, this method is not 100% accurate. Target is not medically examining their customers nor they are taking information from local pregnancy centres.
With such predictions, there would be at least 1-2% bleed. Target might end up sending coupons to people who are not pregnant. May be someone purchased these items for a friend of theirs. But with 98% accuracy, Target can increase its sales and its profits with this prediction.
This became quite controversial when a teenager’s dad found out that his daughter was pregnant based on the postal mails received from Target. This girl hadn’t broke the news even to her dad until then!
Watch this news clip on YouTube to know more. You can also read this article on Time.com – How Target Knew a High School Girl Was Pregnant Before Her Parents Did.
Predictive Analytics in Fraud Detection Systems
Individual criminals and fraudsters always leave fingerprints behind. They do not know what is the kind of fingerprints they shouldn’t leave. When the data of millions of honest people are processed and then if the data of thousands of criminals are processed, there will be certain patterns common with the criminals.
For example, a cyber criminal might use a proxy network, login into certain public internet cafes, send emails at certain times, make calls to certain individuals in a certain pattern. There is no way to randomize this data and no way to have a poker face, online. Note that this is strictly an imaginary scenario. In the real world, there might be a lot more parameters.
If this is the common trend between all cyber criminals and if a random person who is not a suspect yet, exhibits such a behaviour, there is a chance that he/she is going to commit cyber fraud.
This is almost like the movie Minority Report. There could be a pre-crime department in the dystopian future where people are convicted for the frauds they are yet to commit. That’s why every government in the world is so interested in the data of its citizens.
Predictive analytics also plays a huge role in banking and financial fraud detection systems. Most of the systems are hybrid where there is some level of human intervention. As predictive analytics technology becomes better, the percentage of human intervention required becomes less over time.
If you are a credit card user, you might have got a call from your bank when there was a transaction that was suspicious. Such a transaction would have been flagged by a computer using predictive analytics in real time. Because it is impossible for every transaction to be cross-verified manually.
The Future Predictive Analytics in Digital Marketing
Now that you have understood how predictive analytics works and how it can be applied in other areas, let us have a look at the possible applications of predictive analytics in Digital Marketing.
As of now, there are no standard systems established for predictive analytics in digital marketing. Some startups are trying to develop products that can be released in the market, but it is going to take at least a few years before we see revenue predictions inside an analytical tool.
As machine learning algorithms improve, most of the power of predictive analytics will impact end consumers without their knowledge.
For example, Google AdSense has been working on its own ad delivery algorithms to make sure that web masters get the maximum CTR. Google AdWords automatically finds out the ad copy that gets the maximum CTR and starts delivering it more than others.
Email marketing tools will eventually upgrade their systems to make more intelligent and result oriented decisions. For example, based on the history of email opens, one can predict the time frames where a subscriber is most likely to open an email. Instead of sending mass broadcast emails to all subscribers, an email marketing tool using predictive analytics can send emails at specific times customized for each subscriber, to help with higher open rates. Because people usually pay attention to the most recent emails after they open their email Inbox.
Here’s how it might work: Subscriber A will get the same email at morning 10.30 AM and Subscriber B will get the same email at evening 5 PM on a given day. It wouldn’t be rocket science to find out that Subscriber A usually checks email inbox in the morning and Subscriber B usually checks inbox in the evening.
Inside Sales and Lead Scoring Systems can benefit greatly from Predictive Analytics. Sales CRMs usually show a lead score to indicate hot prospects. But the lead scoring systems depend a lot on the setup from the tech and marketing team to predict scores accurately.
With predictive analytics, the model can predict which leads are likely to convert into buyers. If they are highlighted in the sales CRM, the sales agents will only call the prospects that are highly likely to convert into buyers. They will save time in following up with leads who might never buy and just waste the sales person’s time.
Customer Education and Support can be improved with predictive analytics. In most of the SaaS products, only a small percentage of users will reach out to the support department with their issues. In many cases, what they need is customer education. Rest of the users, who do not find a solution, will eventually stop using the product.
If we can predict the set of actions that people do before cancelling their subscription, an automation system can reach out to the customer with solutions before they send a support ticket or think of cancelling. For example, if people find it difficult to do a certain task with a software, we can send an email with further information on how to execute the task.
Conclusion
I hope this article made you realize that predictive analytics is a subject that you cannot ignore. However, we are way ahead of its time when it comes to practical applications. There are so many open loops with digital marketing that predictive analytics would be the last one to be mastered.
Most of the businesses do not even have a proper funnel setup yet and 1000s of businesses are still stuck to traditional marketing. They haven’t even moved to digital marketing yet. So it would be a long time before the true power of predictive analytics is unleashed in the field of digital marketing.
Predictive analytics is a double edged sword. It would raise a lot of privacy concerns especially when the prediction is done on a micro level. Macro level predictive analytics would deal with trends and patterns of data and it would not affect an individual. Just like Google Trends takes data from millions of its users, but doesn’t reveal the search patterns of a single user, macro predictive analytics will always respect individual’s privacy and still get the most out of data for the general good of the human race.
Things can get really messy when predictive analytics starts predicting the behaviour of individual people. Target’s case study shows us that people can get really concerned and disturbed when privacy is invaded on such level.
So even if I can predict that you might buy one of my courses after reading this article, I better not send you a customized offer on your email with a subject like: Are you planning to buy the 100-day blogging Course? Because you just might freak out!
I hope you enjoyed reading this article.
Do leave a comment with your thoughts and share it!